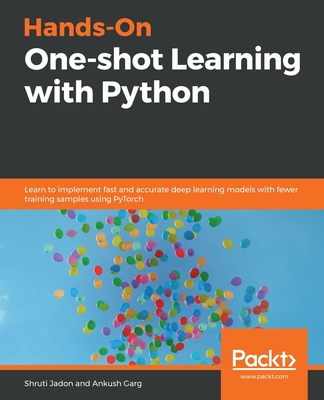
Hands-On One-shot Learning with Python
內容描述
Key Features
Learn how you can speed up the deep learning process with one-shot learning
Use Python and PyTorch to build state-of-the-art one-shot learning models
Explore architectures such as Siamese networks, memory-augmented neural networks, model-agnostic meta-learning, and discriminative k-shot learning
Book Description
One-shot learning has been an active field of research for scientists trying to develop a cognitive machine that mimics human learning. With this book, you'll explore key approaches to one-shot learning, such as metrics-based, model-based, and optimization-based techniques, all with the help of practical examples.
Hands-On One-shot Learning with Python will guide you through the exploration and design of deep learning models that can obtain information about an object from one or just a few training samples. The book begins with an overview of deep learning and one-shot learning and then introduces you to the different methods you can use to achieve it, such as deep learning architectures and probabilistic models. Once you've got to grips with the core principles, you'll explore real-world examples and implementations of one-shot learning using PyTorch 1.x on datasets such as Omniglot and MiniImageNet. Finally, you'll explore generative modeling-based methods and discover the key considerations for building systems that exhibit human-level intelligence.
By the end of this book, you'll be well-versed with the different one- and few-shot learning methods and be able to use them to build your own deep learning models.
What you will learn
Get to grips with the fundamental concepts of one- and few-shot learning
Work with different deep learning architectures for one-shot learning
Understand when to use one-shot and transfer learning, respectively
Study the Bayesian network approach for one-shot learning
Implement one-shot learning approaches based on metrics, models, and optimization in PyTorch
Discover different optimization algorithms that help to improve accuracy even with smaller volumes of data
Explore various one-shot learning architectures based on classification and regression
Who this book is for
If you're an AI researcher or a machine learning or deep learning expert looking to explore one-shot learning, this book is for you. It will help you get started with implementing various one-shot techniques to train models faster. Some Python programming experience is necessary to understand the concepts covered in this book.
目錄大綱
Introduction to One-shot Learning
Metrics-Based Methods
Models-Based Methods
Optimization-Based Methods
Generative Modeling-Based Methods
Conclusion and Other Approaches
作者介紹
Shruti Jadon is currently working as a Machine Learning Software Engineer at Juniper Networks, Sunnyvale and visiting Researcher at Rhode Island Hospital (Brown University). She has obtained her master's degree in Computer Science from University of Massachusetts, Amherst. Her research interests include deep learning architectures, computer vision, and convex optimization. In the past, she has worked at Autodesk, Quantiphi, SAP Labs, and Snapdeal.
Ankush Garg is currently working as a Software Engineer in the auto-translation team at Google, Mountain View. He has obtained his master's degree in Computer Science from the University of Massachusetts, Amherst and Bachelor's at NSIT, Delhi. His research interests include language modeling, model compression, and optimization. In the past, he has worked as a Software Engineer at Amazon, India.